Predictive maintenance, a term increasingly resonant in the modern industrial lexicon, represents a pivotal shift in how businesses approach equipment upkeep. This maintenance strategy, distinct yet often conflated with preventive maintenance, hinges on the principle of foresight. Unlike preventive maintenance, which follows a scheduled approach, predictive maintenance is about anticipating maintenance needs before they become critical.
Predictive Maintenance Definition and Overview
At its core, predictive maintenance is a proactive strategy that utilizes real-time data, advanced analytics, and IoT (Internet of Things) technologies to predict equipment failure before it happens. This method relies heavily on sensors and condition monitoring techniques, such as vibration analysis and oil analysis, to continually assess the condition of equipment. By harnessing machine learning algorithms and predictive analytics, it provides actionable insights, allowing maintenance teams to address issues before they lead to equipment failure or unplanned downtime.
How Predictive Maintenance Differs from Preventive Maintenance
While both preventive and predictive maintenance aim to avoid equipment failure and reduce downtime, their approaches are fundamentally different. Preventive maintenance is scheduled based on time or usage, akin to a car’s regular service intervals. Predictive maintenance, however, is condition-based; it’s driven by data and real-time monitoring, not a fixed schedule.
This key distinction not only makes predictive maintenance more efficient but also reduces the occurrence of unnecessary maintenance activities, thereby cutting maintenance costs significantly.
The Importance of Predictive Maintenance in Modern Industries
Role in Reducing Maintenance Costs and Minimizing Downtime
The implementation of a predictive maintenance program can have a profound impact on reducing maintenance costs. By identifying potential issues before they escalate, businesses can avoid the hefty expenses associated with unplanned downtime. Reactive maintenance, which occurs after equipment failure, often leads to costlier repairs and longer downtime. Predictive maintenance, in contrast, allows for maintenance to be performed only when necessary, thus optimizing maintenance scheduling and resource allocation.
Impact on Equipment Reliability and Maintenance Management
Predictive maintenance isn’t just about preventing failure; it’s also about enhancing the reliability and longevity of every piece of equipment. By monitoring equipment conditions in real time and utilizing IoT sensors, predictive maintenance programs ensure that machines are operating optimally. This reliability is crucial for industries where equipment failure can have significant repercussions, such as in oil and gas or manufacturing sectors.
Additionally, the integration of predictive maintenance into maintenance management systems transforms how maintenance teams operate. It shifts the focus from reactive measures to a more strategic, data-driven approach. This change not only improves the efficiency of maintenance activities but also contributes to a broader understanding of maintenance and reliability within the organization.
H2: How Does Predictive Maintenance Work?
Sensors and Condition Monitoring
Predictive maintenance heavily relies on the utilization of IoT (Internet of Things) and real-time data, which fundamentally transforms maintenance strategies. Key to this approach are sensors placed on equipment, collecting data points like temperature, vibration, and acoustics. This data is crucial for condition monitoring, enabling the maintenance team to track the health of each piece of equipment continuously.
Examples of how this works in practice include vibration analysis and oil analysis. Vibration analysis detects anomalies in the operational patterns of machinery, often indicative of issues like imbalance, misalignment, or bearing faults. Similarly, oil analysis can reveal contaminants or metal particles that suggest wear and tear in machinery. These condition-based maintenance techniques allow for early detection of potential equipment failure, making predictive maintenance a critical component in modern maintenance management.
Predictive Maintenance Technologies and Solutions
The integration of machine learning and AI in predictive maintenance is revolutionary. These technologies analyze the vast amount of data collected by sensors, identifying patterns and anomalies that might be imperceptible to the human eye. Predictive analytics plays a significant role here, helping to forecast when maintenance should be performed, thereby reducing the likelihood of unexpected equipment failure. This capability not only enhances the efficiency of maintenance activities but also aligns closely with evolving maintenance strategies that prioritize data-driven decision-making.
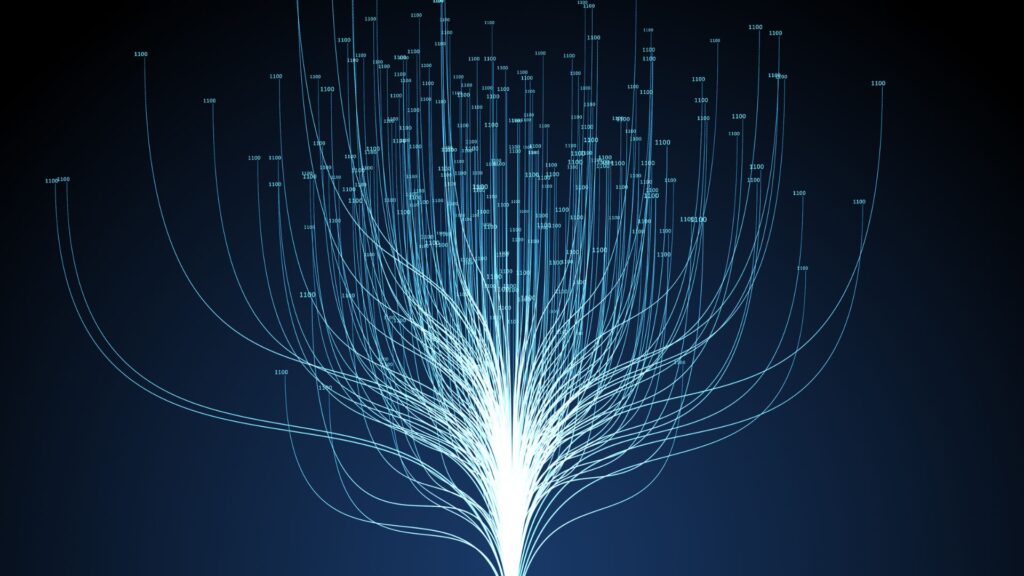
Predictive Maintenance Programs and Implementation
Implementing a predictive maintenance program involves several steps, starting from the installation of sensors to the integration of analytics software. It’s essential to have a robust maintenance team and management system in place to interpret data and act on the insights provided. The success of such a program hinges on the team’s ability to understand and utilize the data effectively, which often necessitates training in new technologies and processes. A well-executed predictive maintenance program becomes a cornerstone of modern maintenance management, ensuring equipment reliability and operational efficiency.
H2: Benefits of Predictive Maintenance
Reducing Maintenance Costs
One of the primary benefits of predictive maintenance is its ability to significantly reduce unplanned downtime and associated maintenance costs. Case studies across various industries have shown that predictive maintenance, when properly implemented, can foresee equipment failure, allowing for repairs to be scheduled at the most opportune times. This proactive approach contrasts starkly with reactive maintenance, where repairs occur after a failure, often leading to more extensive damage and higher costs. Predictive maintenance, with its focus on early detection, ensures that maintenance is performed only when necessary, optimizing resource use and minimizing disruptions to operations.
Enhancing Equipment Life and Reliability
Predictive maintenance plays a pivotal role in extending the life of equipment and improving its reliability. By continuously monitoring the condition of equipment and addressing issues before they escalate, predictive maintenance helps avoid the severe damage that can result from equipment failures. This approach is more nuanced than traditional preventive maintenance strategies, which are based on time or usage intervals rather than actual equipment condition. By focusing on the real-time health of the equipment, predictive maintenance ensures that maintenance activities are more targeted and effective.
Integrating Predictive Maintenance with IoT and Big Data
The integration of predictive maintenance with IoT and big data is a game-changer. IoT sensors provide a constant stream of data, which, when combined with big data analytics, offers unprecedented insights into equipment performance. This integration allows for more accurate predictions and smarter maintenance decisions. Algorithms process this data in real-time, identifying trends and anomalies that indicate potential problems. As a result, maintenance teams can make informed decisions, schedule maintenance more effectively, and avoid the pitfalls of both unplanned downtime and unnecessary maintenance activities.
Predictive Analytics: The Impact and Effectiveness
Evaluating the Economic Impact
The economic benefits of predictive maintenance are substantial. According to Deloitte, a non-optimized maintenance strategy can lead to a reduction in production capacity of 5 to 20%. On the other hand, predictive maintenance can significantly cut down the costs associated with unplanned downtime, which, as per industry estimates, cost industrial manufacturers around 45 billion euros annually. By reducing these losses, predictive maintenance not only saves money but also contributes to smoother and more reliable production processes.
Technological Advancements and Challenges
The core of predictive maintenance lies in its technological foundation. The use of IoT devices and sensors allows for the continuous monitoring of equipment, generating vast amounts of data. This data, when analyzed through AI and machine learning algorithms, provides insights into the potential failure points of machinery. However, the challenge lies in effectively managing and interpreting this data. Developing accurate predictive models requires significant expertise in data analytics and a deep understanding of machine behavior.
The Future of Maintenance: Predictive and Beyond
Emerging Trends in Predictive Maintenance Market
The predictive maintenance market is experiencing significant growth, driven by the evolving role of AI and machine learning. These technologies are becoming increasingly sophisticated, allowing for more accurate predictions and efficient maintenance processes. According to Next Move Strategy Consulting, the global predictive maintenance market, valued at 4.5 billion U.S. dollars in 2020, is projected to reach 64.3 billion U.S. dollars by 2030. This exponential growth highlights the rising adoption of predictive maintenance across various industries.
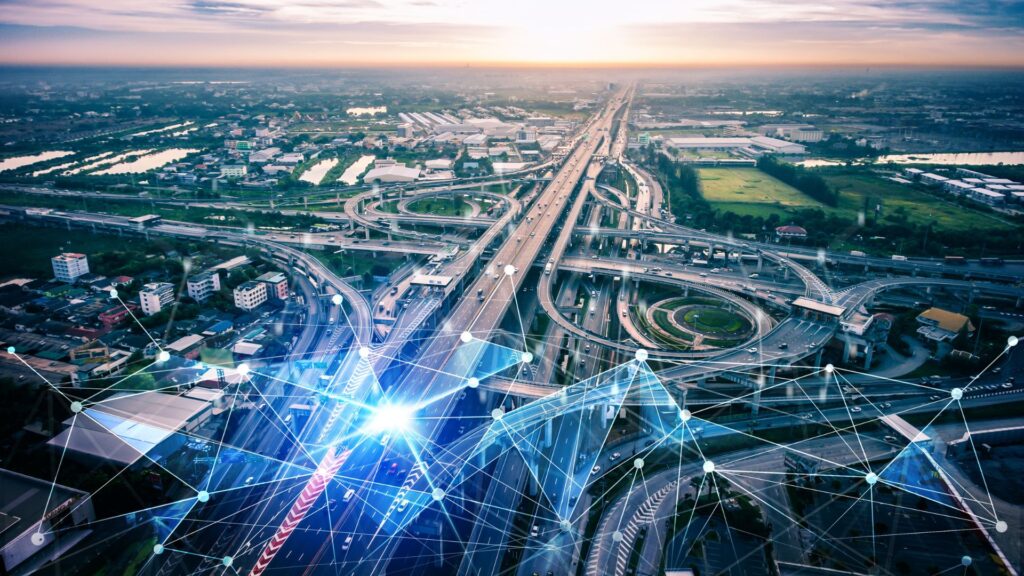
AI and machine learning are at the forefront of this evolution, offering enhanced capabilities in analyzing large datasets to predict equipment failure. This progression leads to more nuanced and effective maintenance strategies, where decisions are data-driven and based on real-time insights.
Predictive Maintenance as Part of a Larger Maintenance Strategy
Predictive maintenance is increasingly being recognized as a crucial component of a comprehensive maintenance strategy. It complements other types of maintenance, such as preventive and condition-based maintenance, by providing a more detailed understanding of equipment health. This synergy enables organizations to develop a more effective maintenance plan, balancing different approaches to achieve optimal results.
For example, while preventive maintenance is scheduled at regular intervals, predictive maintenance can be used to fine-tune these schedules based on actual equipment condition. This integration not only enhances the overall effectiveness of maintenance activities but also contributes to significant cost savings and efficiency improvements.
Example of Market Size: A real-world example of the growing significance of predictive maintenance can be seen in the road maintenance market. This market, driven by the need for infrastructure development and increasing traffic congestion, is adopting advanced technologies like AI, machine learning, and IoT. The use of predictive maintenance in road maintenance ensures efficient and timely upkeep of roads, reducing the risk of accidents and prolonging the lifespan of road infrastructure.
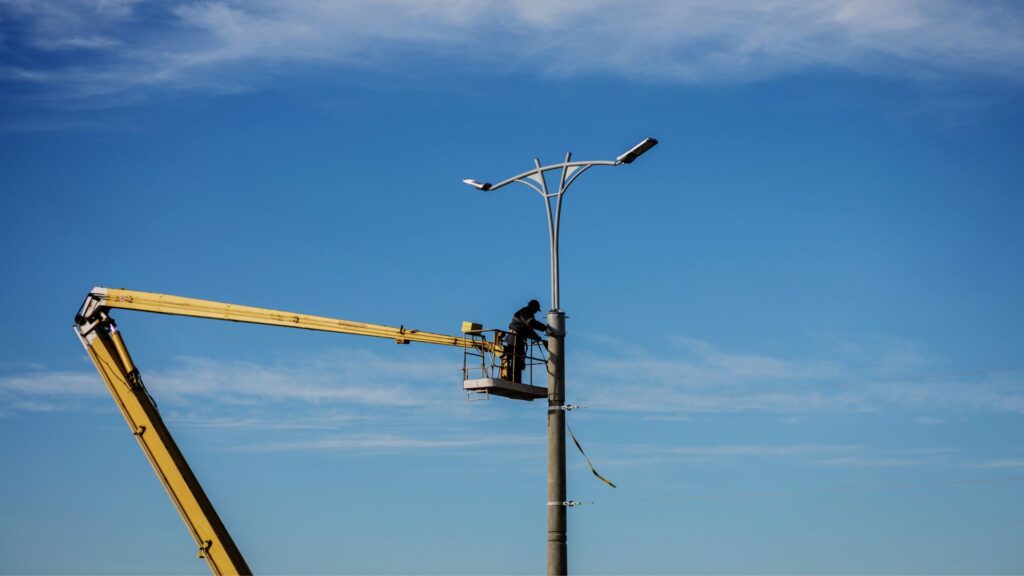
The road maintenance market itself is poised for considerable growth, with advanced data analytics and predictive maintenance techniques being key trends. The integration of these technologies in maintenance operations signifies a broader shift towards smarter, more efficient maintenance practices across industries.
Conclusion
Predictive maintenance, a cornerstone of Industry 4.0, represents a significant shift in how industries approach equipment maintenance. By harnessing the power of data analytics, IoT, and advanced technologies like AI and machine learning, predictive maintenance transcends traditional maintenance methods, offering a more efficient, cost-effective, and reliable approach. It not only helps in averting equipment failures and reducing downtime but also plays a crucial role in enhancing the overall operational efficiency of industries. The integration of predictive maintenance into industrial practices is not just a trend but a necessity in the modern, data-driven world. As we move forward, the adoption and refinement of predictive maintenance strategies, supported by robust ModelOps frameworks, will continue to be a key factor in the success and competitiveness of industries globally.
References
- Liguori, G. (2022, March 23). Predictive maintenance in industry 4.0: applications and advantages. LinkedIn. https://www.linkedin.com/pulse/predictive-maintenance-industry-40-applications-giuliano-liguori/
- Deloitte. (n.d.). Maintenance strategy and its impact on production capacity. Deloitte Research. https://www2.deloitte.com/global/en/pages/manufacturing/articles/maintenance-strategy-production-capacity.html
- Industrial Internet of Things (IIoT) Market Size. (2025). Market Analysis Report. https://www.researchandmarkets.com/reports/4826601/industrial-internet-of-things-iiot-market-size
- ModelOp Center v3.0. (n.d.). ModelOp. https://www.modelop.com/modelop-center/
Author
-
Johnny Wu
Specializing in SEO and digital marketing with a passion for enhancing online visibility. With over a year in the IoT industry, I focus on advancing roadway IoT and AI technologies to improve traffic management and road safety.